Artificial Intelligence and Machine Learning (AI/ML) are becoming cornerstones for business operational efficiency and innovation. For technology and business leaders, this isn't just about tapping into new tech trends; it's about integrating these tools in a manner that's strategic, compliant, and aligned with business objectives. Effective governance of AI/ML ensures its ethical application, transparency, and adherence to regulatory standards. This article offers a look at the stages of AI/ML adoption, alongside the governance practices that should accompany each phase. The aim is to present a working framework, facilitating informed decision-making as organizations increasingly rely on AI/ML. By understanding the nuances of AI/ML governance, businesses can maximize benefits while mitigating potential risks.
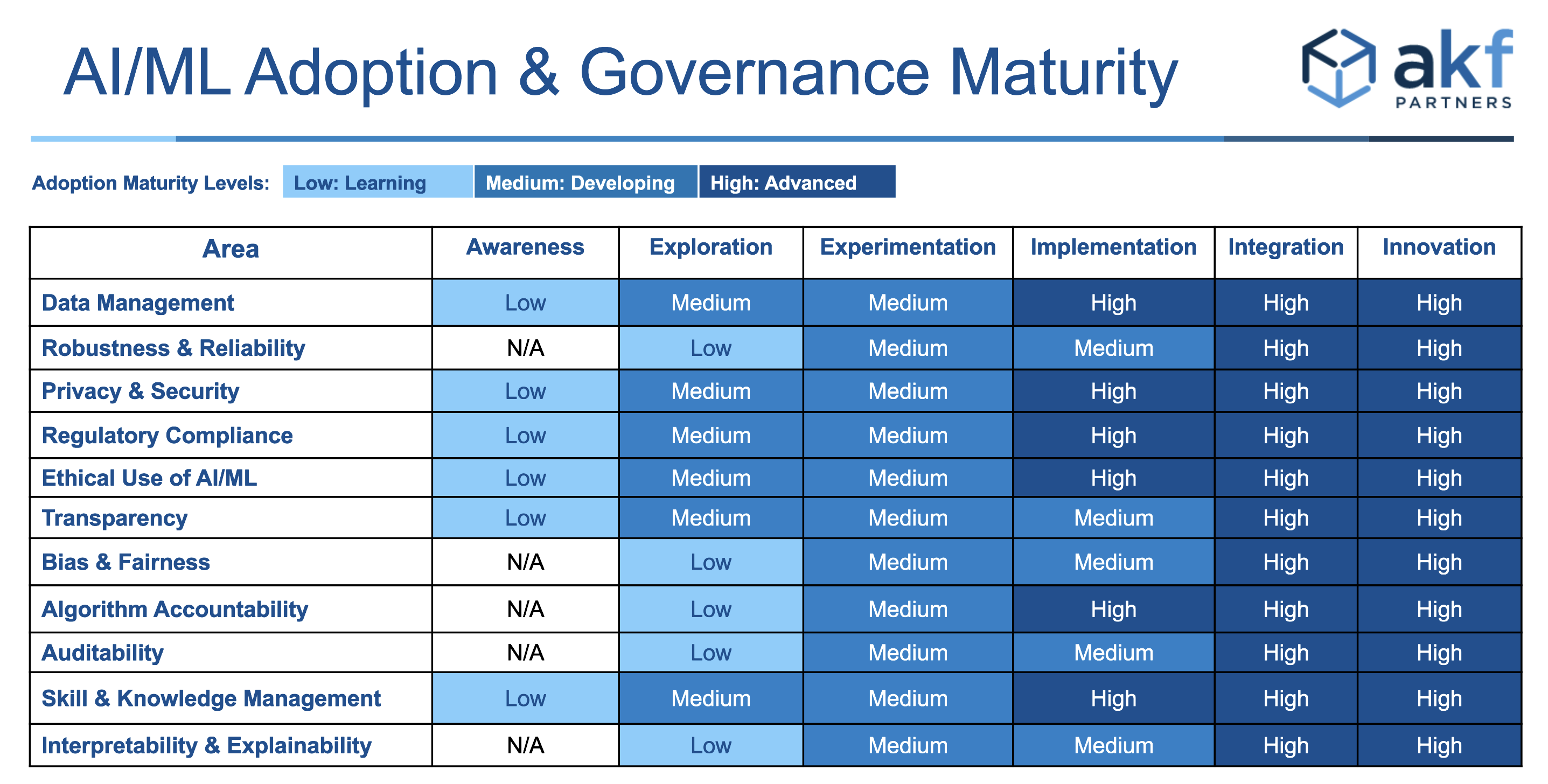
AI/ML Adoption Stages
In today's rapidly evolving technological landscape, AI/ML stands out as a transformative force reshaping industries and business models. Understanding the progression of AI/ML adoption in a corporate setting is not just a luxury but a necessity for forward-thinking executives. As organizations embark on this multifaceted journey, delineating the adoption stages provides a roadmap and shared language. This roadmap not only demystifies the transition phases but also offers strategic insights, enabling leaders to navigate the intricacies of integration, manage potential challenges, and harness the full potential of AI/ML to drive innovation and growth.
- Awareness: At this initial stage, a company begins to recognize the transformative potential of AI/ML. While there might not be any hands-on implementation yet, there's a burgeoning curiosity. Firms start to grasp the broader implications of AI/ML and monitor how competitors leverage these technologies, laying the groundwork for a strategic response in the future.
- Exploration: Transitioning from mere recognition, companies enter a phase of tangible action. They initiate pilot projects or proofs of concept to test the waters and understand AI/ML's applicability to their specific needs. Concurrently, there's a focus on upskilling the workforce, ensuring they have the preliminary tools and knowledge to navigate the impending AI/ML-driven changes.
- Experimentation: Riding on the success of early forays into AI/ML, companies broaden their scope of experimentation. They explore the application of AI/ML across various business areas, be it marketing, operations, or customer service. This stage, while filled with the excitement of discovery, is also marked by inevitable challenges and setbacks. It serves as a learning curve, refining the company's AI/ML strategies.
- Implementation: Companies have moved past exploratory phases and are ready to embrace AI/ML on a larger scale. Drawing from lessons learned during the experimentation phase, AI/ML technologies are applied more extensively within the organization. This stage sees a surge in resource allocation, both in terms of capital and manpower, as companies begin to witness tangible benefits from AI/ML adoption.
- Integration: AI/ML technologies are no longer stand-alone projects or isolated initiatives. They become deeply embedded within the company's operations, driving efficiency, and consistently offering significant benefits. The organization's proficiency in AI/ML positions it favorably within its industry, making it a beacon for others to emulate.
- Innovation: At the zenith of AI/ML adoption, companies don't just use AI/ML; they push its boundaries. They are not only adopting existing technologies but are at the forefront of AI/ML innovation, setting industry trends and standards. Their expertise and pioneering efforts in AI/ML position them as undisputed market leaders, setting benchmarks for excellence and innovation in the domain.
In understanding these stages, technology and business leaders are better positioned to anticipate the challenges, allocate resources effectively, and align AI/ML adoption with broader corporate goals.
AI/ML Governance Areas
As AI/ML technologies steadily infiltrate the core of modern business operations, their governance moves from being a peripheral concern to a central aspect of corporate strategy. For executives overseeing the integration of AI/ML into business processes, governance goes beyond being merely topical—it stands as the backbone of ethical and sustainable technology adoption. The incorporation of AI/ML isn't a straightforward endeavor; it brings to the fore a multitude of complexities, ranging from meticulous data management to the imperative of ethical application. As such, it's not only advantageous but paramount to delineate and establish robust governance structures across critical domains. These structures will act as guiding pillars, ensuring that AI/ML adoption is not only technologically proficient but also ethically sound, legally compliant, and strategically aligned with an organization's overarching vision.
- Data Management: This governance domain revolves around the holistic management of data within an organization. It goes beyond mere data storage and retrieval. Effective data management ensures rigorous protocols for data acquisition, emphasizes the quality and accuracy of data, and prioritizes the safety and confidentiality of information. It underscores the importance of aligning data practices with legal requisites and corporate ethics. A well-governed data management system safeguards privacy while fostering a reliable data foundation for AI/ML applications.
- Robustness & Reliability: Ensuring the dependability of AI/ML systems is critical to their successful integration. Governance in this area focuses on establishing measures that guarantee systems function predictably and in line with expectations. By prioritizing both robustness and reliability, businesses can reduce the risk of unforeseen system failures, safeguarding both operational continuity and stakeholder confidence in AI-driven initiatives.
- Privacy & Security: With AI/ML systems often entrusted with vast volumes of sensitive and personal data, governance prioritizes the establishment of stringent security measures. The focus isn't just on preventing unauthorized access but also on ensuring the integrity and confidentiality of the data. Governance mechanisms in this domain safeguard personal data and maintain system resilience against potential threats.
- Regulatory Compliance: AI/ML applications don't operate in a vacuum. Various industries are governed by specific rules and regulations that dictate how AI/ML can be deployed. Effective governance means continuously updating systems and processes to be in line with these regulatory standards, thereby minimizing legal liabilities and reinforcing the organization's commitment to lawful and responsible technology adoption.
- Ethical Use of AI/ML: While regulations provide a clear framework for AI/ML deployment, ethical governance goes a step further. It ensures that AI/ML applications respect broader societal values, human rights, and cultural norms. It's about guaranteeing that the technology is used in a manner that is morally justifiable and doesn't compromise human dignity or societal harmony.
- Transparency: The "black box" nature of many AI/ML models can be a barrier to trust. Governance in this area emphasizes the need for clarity regarding how these systems make decisions. By being open about the operations and logic behind AI/ML systems, organizations can foster trust, ensuring that consumers, employees, and other stakeholders are well-informed and can make knowledgeable decisions.
- Bias & Fairness: AI/ML models are only as good as the data they're trained on. Governance in this domain is dedicated to detecting and rectifying biases in AI models. It's about ensuring that AI-driven decisions are equitable, impartial, and don't inadvertently reinforce existing stereotypes or societal inequities.
- Algorithm Accountability: As powerful as AI/ML models are, they aren't infallible. Governance mechanisms here define clear lines of responsibility regarding AI/ML outcomes. By knowing who or what is accountable for specific AI-driven results, it becomes feasible to address any deviations promptly and effectively.
- Auditability: To ensure the sustained performance and ethical operation of AI/ML systems, regular audits are crucial. Governance in this area stresses the importance of periodic checks that validate the accuracy, fairness, and reliability of AI models. Such audits reinforce the system's integrity, ensuring its alignment with established standards and norms.
- Skill & Knowledge Management: To harness the true potential of AI/ML, an adept workforce is non-negotiable. This domain focuses on the continuous development of employee expertise in AI/ML, addressing both foundational knowledge and emerging trends. Through regular training, upskilling, and fostering a culture of continuous learning, organizations ensure that they remain at the forefront of technological advancements, while also guaranteeing the responsible and optimized use of AI/ML in their operations.
- Interpretability & Explainability: As AI/ML systems become increasingly complex, their decision-making processes can appear opaque. This domain prioritizes the clarity and accessibility of AI/ML outputs. It emphasizes the development and deployment of models that, while intricate, can be broken down and communicated in a manner that stakeholders can grasp. Ensuring that the reasoning behind AI/ML-driven decisions is both intelligible and transparent fosters trust, facilitates regulatory compliance, and promotes informed decision-making.
By addressing each of these governance areas, companies can ensure that their organization's AI/ML adoption is not only technologically sound but also ethically responsible and aligned with best practices.
The Interplay of AI/ML Adoption and Governance Maturity
As companies delve deeper into AI/ML adoption, each phase brings its set of governance challenges. This progression isn't linear—it's layered, with each stage building on the previous. For executives, understanding the relationship between the adoption phase and its corresponding governance requirements is a practical necessity. It ensures that governance measures are introduced appropriately and remain effective as AI/ML initiatives expand. In this section, we'll examine the specific governance focus areas pertinent to each adoption stage and discuss their practical implications for businesses.
- Awareness: At this foundational stage, the emphasis lies primarily on Data Management. As organizations begin to explore AI/ML's potential, understanding the data landscape is paramount. Without a comprehensive view of data sources, quality, and compliance, any subsequent AI/ML initiatives could be built on shaky ground. Likewise, an early emphasis on Privacy & Security means companies begin their AI/ML journey with a commitment to data protection—a crucial foundation for trust.
- Exploration: With pilot projects underway, there's a marked increase in hands-on interaction with AI/ML technologies. This raises the importance of Skill & Knowledge Management. Training the workforce and setting initial guidelines become crucial. As prototypes are developed, Regulatory Compliance and Transparency become significant. These ensure that even early-stage projects respect legal requirements and that stakeholders have visibility into AI-driven processes.
- Experimentation: As organizations cast a wider net with AI/ML across different business areas, the risk and potential impact of biases increase. This stage thus emphasizes Bias & Fairness. Ensuring that AI models are free from unintentional biases helps prevent skewed outcomes. Additionally, as projects diversify, having checks in place for Algorithm Accountability becomes necessary. This ensures that there's clarity on responsibility should an AI model produce undesired results.
- Implementation: Now, with AI/ML becoming a significant part of operations, the stakes are higher. Governance areas like Auditability and Interpretability & Explainability come to the fore. Regular audits ensure that AI models remain accurate and reliable. Moreover, as AI-driven decisions become commonplace, their reasoning must be explainable to stakeholders, emphasizing the need for clear model interpretations.
- Integration: With AI/ML fully embedded in operations, governance requires a holistic approach. Every facet, from Robustness & Reliability to Ethical Use, must be meticulously addressed. It's about ensuring that the technology not only works seamlessly but also upholds the highest standards of ethics and compliance.
- Innovation: In this final stage, businesses are setting industry trends. The governance focus here is comprehensive, reinforcing all the earlier stages but with an emphasis on continual improvement and leading by example. At this stage, businesses aren't just ensuring their AI models are compliant; they're setting the standards for what responsible AI/ML looks like in their industry.
The relationship between AI/ML adoption and governance maturity is dynamic, and by recognizing the nuances of each stage, executives can anticipate challenges, uphold best practices, and guide their organizations to leverage AI/ML responsibly and effectively.
Conclusion
The integration of AI/ML into the modern business landscape is not merely about leveraging technology for operational advantages. It represents a paradigm shift in how businesses operate, make decisions, and evolve in an increasingly data-driven environment. For technology and business leaders, understanding the stages of AI/ML adoption and the corresponding governance maturity is not just advisable—it's imperative. It ensures that as organizations climb the ladder of AI sophistication, they do so with the guidance of a robust framework, minimizing risks and maximizing benefits. By focusing on governance as a parallel trajectory to adoption, businesses can uphold their commitments to stakeholders, maintain trust, and position themselves as leaders in a world where AI/ML is rapidly reshaping industries. This dual journey of technological advancement and ethical governance will undoubtedly define the successful enterprises of the future.
As a reminder, the areas of governance and adoption stages outlined in this article serve as a general framework for AI/ML implementation. They provide a broad overview based on common practices and challenges. Organizations should be aware that specific requirements or legal obligations may vary and are encouraged to consult relevant experts or legal counsel to ensure comprehensive compliance and effective implementation.
Related Posts
</br>