For firms focused on technology-driven acquisitions, artificial intelligence (AI) and machine learning (ML) are becoming central to evaluating the potential of target companies. These technologies can unlock operational efficiencies, automate complex tasks, and enable data-driven decision-making. However, assessing AI/ML solutions during due diligence requires more than just recognizing their technical capabilities—it demands an understanding of how they perform in practice, how they can scale, and how they might evolve in the future.
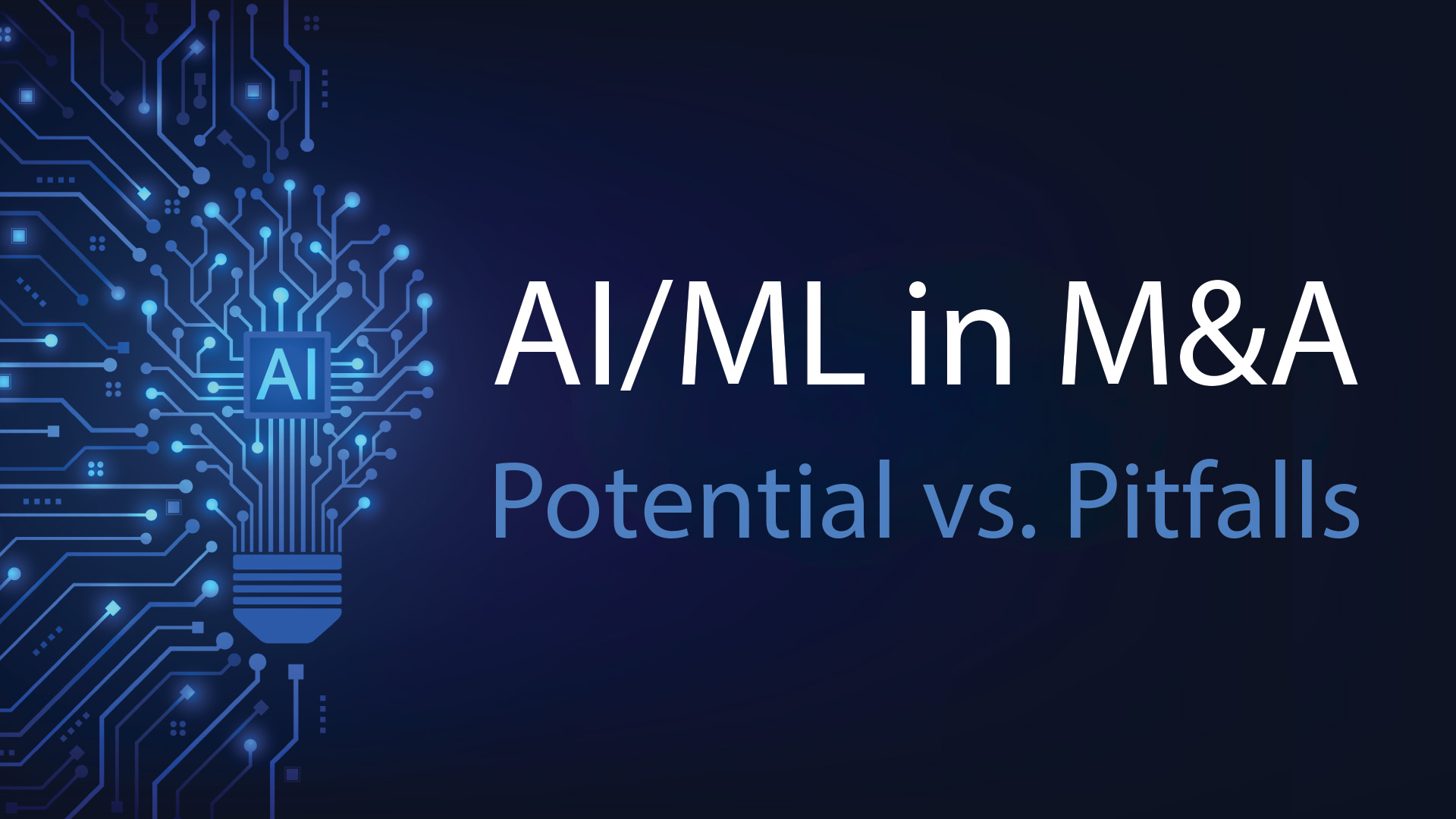
One challenge in evaluating AI/ML is the rapid pace of innovation. A solution that offers a competitive advantage today may quickly become standard as more sophisticated AI tools, like large language models (LLMs), make similar functionality widely available. For example, AI models that rely on proprietary data for training could be replaced by general-purpose systems that deliver similar value through advanced prompt engineering. This shift could reduce the long-term value of AI/ML assets unless they offer a sustainable, differentiating factor.
This article explores how PE firms can assess AI/ML capabilities during tech diligence, with a focus on evaluating the maturity, scalability, and potential risks of these solutions. By understanding both the current application and future implications, firms can make more informed decisions about the real value AI/ML brings to their acquisitions.
Understanding AI/ML Maturity in Acquisition Targets
When evaluating a company’s AI/ML capabilities, it’s important to look beyond surface-level claims and assess how developed, integrated, and scalable these technologies are. AI/ML maturity refers to how well these solutions are built to provide real value and sustain future growth. A careful evaluation should focus on several key factors that reveal whether the AI/ML capabilities are positioned to drive meaningful business impact or if they are still in early, unproven stages.
Technology Architecture
A well-designed AI/ML system should have a solid architecture that allows for scaling and flexibility as the business grows. This includes infrastructure that supports efficient model development, deployment, and ongoing management. An AI/ML system that can handle increasing data volumes and complexity without needing frequent rework is a sign of maturity. Firms should also assess whether the technology is cloud-based or on-premise, as this will impact how easily the system can be expanded or adapted in the future.
Integration with Existing Systems
For AI/ML to deliver real value, it needs to be seamlessly integrated into the company’s core systems and workflows. Solutions that sit in isolation or are only used in limited pilots are less likely to produce sustained business benefits. Mature AI/ML implementations are tightly connected to essential platforms like customer relationship management (CRM) or enterprise resource planning (ERP) systems. This level of integration ensures that AI/ML insights can be easily translated into actions, making the technology a core part of the company’s operations.
Data Availability and Quality
AI/ML models depend on data to function effectively. The quality, consistency, and accessibility of the company’s data are critical in determining whether the AI/ML technology is robust enough to support business objectives. A firm needs to assess whether the company has access to proprietary datasets that could offer a competitive advantage or if the data is widely available and easily replicated by others. Additionally, the maturity of data governance practices—such as how well data is managed, cleaned, and secured—provides insight into how reliable the AI/ML models will be in the long term.
Team Expertise
The expertise of the team behind the AI/ML initiatives is another important indicator of how advanced the company’s capabilities are. Skilled data scientists, machine learning engineers, and domain experts are essential for developing, maintaining, and refining AI/ML solutions. A strong team is also better equipped to adapt to the fast-evolving AI landscape and ensure that models continue to deliver value as the technology and business needs change. Evaluating the team’s experience and track record can help determine whether the AI/ML capabilities are built on a solid foundation.
Use of Proprietary vs. Open-Source Technology
It’s also valuable to understand whether the company relies on proprietary AI/ML solutions or utilizes open-source technologies. Proprietary models can sometimes offer a competitive edge, particularly if they’re built on unique algorithms or specialized datasets, but they often come with higher maintenance costs and may be harder to scale. Open-source tools can offer more flexibility and lower costs, though they might not always provide the same level of differentiation. A balanced approach, where proprietary elements are used strategically and open-source tools provide operational efficiency, is often a sign of maturity.
Deployment History and Real-World Applications
A strong indicator of AI/ML maturity is whether the company has successfully applied its solutions in real-world scenarios. Firms should look for evidence that AI/ML is actively solving important business problems, improving operations, or driving revenue growth. The longer the AI/ML solutions have been deployed and delivering measurable results, the more likely it is that the technology is well-established and capable of delivering ongoing value. Case studies, metrics, and a history of successful implementations can offer valuable insights into the effectiveness of the company’s AI/ML initiatives.
Evaluating Scalability of AI/ML Solutions
When assessing the AI and machine learning (ML) capabilities of an acquisition target, it’s crucial to evaluate not only how well these technologies work today but also whether they can scale as the business grows. Scalable AI/ML solutions are those that can handle increased data volumes, user demand, and complexity without significant rework or costly upgrades. A solution that works for a smaller operation may break down or lose efficiency when pushed to support a larger enterprise. To make an informed judgment on scalability, firms should consider several factors that indicate whether the AI/ML systems can support long-term growth.
Model Scalability
One of the core aspects of scalability is whether the AI/ML models themselves can handle increasing data and complexity. A mature solution should be able to process larger datasets, train on more complex information, and maintain or improve accuracy as these inputs grow. Firms should assess how the models perform as data scales and whether they are designed to evolve alongside the company’s expanding needs. This includes understanding how often the models require retraining and whether this process is streamlined enough to scale without excessive manual intervention.
Infrastructure and Cloud Readiness
The underlying infrastructure supporting AI/ML is key to ensuring scalability. Cloud-based systems, for example, often provide the flexibility needed to scale quickly and efficiently as demands change. Firms should evaluate whether the AI/ML platform can leverage elastic cloud resources to handle growing workloads without significant delays or increased costs. If the infrastructure is still on-premise or fragmented, scaling AI/ML solutions may require substantial investment in hardware or architectural redesign. Additionally, firms should examine whether the company has adopted scalable storage solutions, as AI/ML systems often require large amounts of data storage.
Compute Power and Cost Efficiency
AI/ML models, especially those involving deep learning or complex algorithms, can be resource-intensive. To scale effectively, these solutions need to be backed by sufficient compute power. Firms should assess whether the company is currently using efficient computing resources, such as GPUs or distributed systems, and whether these resources can scale cost-effectively. A solution that requires disproportionately high compute resources as it scales could become cost-prohibitive, limiting its long-term value. Consider the trade-offs between performance and cost, particularly when evaluating AI/ML systems that will need to scale rapidly in response to business growth.
Automation and Operational Integration
Scalability also depends on how well AI/ML processes are automated and integrated into the broader operations of the company. Solutions that require significant manual tuning, intervention, or custom development at each stage of growth may face bottlenecks that limit scalability. Conversely, AI/ML systems that are designed for automation—such as auto-scaling, automated retraining, and integration into decision-making workflows—are better equipped to scale seamlessly. A scalable AI/ML solution should have clear pipelines for data ingestion, model training, and deployment, all of which should operate with minimal human oversight.
Sustainability of Scalability in a Rapidly Changing AI Landscape
Another factor to consider is how well the AI/ML solution will hold up in the face of rapid technological advances. While a model may seem scalable today, the AI landscape is evolving quickly, particularly with the rise of more powerful large language models (LLMs) and general-purpose AI tools. What may require specialized, proprietary data today could be handled more efficiently by future advancements in LLMs or generalized models that can achieve similar outcomes with less effort. This trend could potentially diminish the need for proprietary solutions, meaning that firms must consider not only the scalability of the current system but also how future developments might shift the scalability equation.
Adaptability to New Business Needs
Beyond technical scalability, firms should also evaluate how adaptable the AI/ML solutions are to changing business requirements. Can the AI/ML systems be repurposed or reconfigured to support new products, markets, or use cases? For example, if a company plans to expand into new regions or industries, will the AI/ML models and infrastructure support these moves without needing extensive redevelopment? Solutions that are flexible and can be easily adapted to new use cases or industries are more likely to deliver sustained value as the company grows and evolves.
Evaluating Model and Technology Selection
Selecting the right AI/ML technology for a specific task is crucial in determining both the effectiveness and scalability of the solution. This process involves complex tradeoffs between factors like cost, speed, and purpose, and requires a nuanced approach to avoid a one-size-fits-all mentality. By evaluating the thought process behind these decisions, firms can better understand the maturity and expertise of the team implementing these technologies.
Tradeoffs in Model Selection
Choosing the appropriate AI/ML model means balancing the complexity of the task with the resources available. For simpler tasks, using a sophisticated model like a large language model (LLM) can be unnecessary and inefficient. Instead, lightweight models such as GPT mini or even traditional machine learning algorithms can offer faster, more cost-effective solutions. The key is selecting a model that aligns with the problem’s requirements without overcomplicating the approach.
Nuanced Model Selection Reflects Expertise
A team’s ability to select the right model shows their level of expertise. If the company defaults to using one model for all tasks, it may indicate a lack of experience. Mature AI/ML teams will understand that different industries and tasks require different approaches. For instance, a financial application may need more sophisticated models than a basic customer service chatbot. The team’s understanding of when and where to apply different models is a key indicator of their strategic thinking.
LLMs vs. Traditional AI/ML Models
LLMs like OpenAI’s ChatGPT are not always the best choice for every task, especially when simpler models like neural networks or regression could deliver faster, more predictable results at a lower cost. However, if training data is limited or the team lacks the expertise to develop and maintain these simpler models, an LLM may still make sense. The decision should be based on the complexity of the task and available resources, not just a desire to use the latest AI technology.
Evaluating the Team's Thought Process
The the thought process behind AI/ML technology selection is at least as important as the decision itself. A team that considers the tradeoffs between different AI/ML models and can clearly explain their decisions demonstrates maturity. Firms should evaluate not only what models are in use but also the reasoning behind those choices, as this reveals the team’s capability to adapt to future challenges and opportunities in AI/ML deployment.
Navigating the Rapid Evolution of AI/ML: From Differentiator to Commodity
The fast progression of AI/ML means that what is considered innovative today can quickly become a standard offering. As generalized AI models and tools, like large language models (LLMs), become more sophisticated, firms need to assess how sustainable a company’s AI/ML advantage really is. A key part of this evaluation is understanding whether the target’s AI/ML solutions are built to retain their edge as the technology landscape shifts and whether the company has a strategy to stay ahead as AI/ML capabilities become more commoditized.
Proprietary Models vs. Generalized AI
Proprietary AI/ML models that once delivered a significant competitive advantage may soon face challenges as generalized AI tools become more powerful and easier to deploy. Firms should evaluate whether the target relies on unique models or datasets that will maintain their value or if they could be replaced by more versatile, off-the-shelf AI solutions. The ability to adapt and innovate as these generalized tools evolve is crucial for maintaining a competitive edge.
First-Mover Advantage and Sustained Innovation
While being an early adopter of AI/ML can provide an initial lead, that advantage can quickly diminish if there’s no plan for ongoing evolution. Firms should assess whether the target has a long-term strategy for staying ahead, rather than just relying on the short-term gains of early adoption. Continuous investment in AI/ML R&D and the ability to pivot as new tools emerge will be key to preserving a first-mover position.
Commoditization and Future-Proofing AI/ML Investments
As AI/ML solutions become more commoditized, the risk of losing differentiation grows. Firms should evaluate how vulnerable the target’s AI/ML offerings are to commoditization and whether the company has strategies in place to mitigate this risk. Building flexibility into AI/ML systems and maintaining a culture of innovation are essential for ensuring that these technologies continue to deliver value as the market evolves.
Common Pitfalls in Evaluating AI/ML Capabilities
Evaluating AI/ML capabilities during due diligence can be complex, and there are several common pitfalls that firms must avoid. Misjudging the true value or sustainability of AI/ML technology can lead to overestimating its potential impact, underestimating risks, or overlooking critical limitations. Recognizing these common traps can help firms make more informed, accurate assessments.
Overhyping AI/ML Potential
One of the most common mistakes is assuming that AI/ML solutions are automatically transformative just because they incorporate advanced technologies. The reality is that not all AI/ML implementations lead to meaningful business impact. Firms should critically assess whether the AI/ML solutions are actually driving value or if they are merely hyped with little real-world application. Look for measurable outcomes, such as revenue generation or operational efficiencies, rather than relying on theoretical benefits.
Ignoring Data Quality and Availability
AI/ML models rely heavily on high-quality, well-organized data. One of the biggest pitfalls is overlooking the data infrastructure that supports these models. A company may have sophisticated algorithms, but if the data is incomplete, poorly structured, or insufficient in volume, the models will likely fail to deliver accurate results. Firms need to evaluate the robustness of the data pipeline, ensuring that the company has access to the right quantity and quality of data to support long-term AI/ML success.
Underestimating Scalability and Maintenance Costs
AI/ML solutions can be resource-intensive, and scaling these models is not always straightforward. A common pitfall is underestimating the cost and effort required to scale AI/ML capabilities as the business grows. This includes both the technical infrastructure required, such as cloud resources or specialized hardware, and the ongoing need for model maintenance and retraining. Firms should evaluate whether the target’s AI/ML infrastructure and budget can handle scaling needs without significant disruption or excessive cost increases.
Overlooking Security Challenges
AI/ML solutions, particularly those handling sensitive or regulated data, present unique security challenges. This is especially true in multitenant environments where multiple customers’ data is processed on shared infrastructure. Firms must assess whether the target has appropriate security measures in place to protect data from breaches, unauthorized access, or misuse. Encryption, secure data storage, and robust access control policies are critical for AI/ML systems that deal with personal, financial, or healthcare-related information. Ignoring these security requirements could lead to vulnerabilities that expose the company to data breaches and compliance risks, especially in highly regulated industries.
Neglecting Governance and Regulatory Requirements
As AI/ML becomes more integral to business operations, regulatory scrutiny is increasing. Governments and regulatory bodies are working to establish clearer guidelines around AI/ML use, especially concerning ethical considerations, data privacy, and fairness. A common pitfall is neglecting to assess whether the company has the proper governance structures in place to ensure compliance with current regulations, as well as the flexibility to adapt to future changes. Firms should evaluate the company’s data governance policies, transparency of AI/ML decision-making processes, and readiness for emerging regulations. Non-compliance or lack of foresight in this area could expose the company to significant legal and reputational risks down the line.
Assessing the Value of AI/ML in Tech Due Diligence
Determining the value of AI/ML capabilities during tech due diligence involves more than just evaluating the sophistication of the technology. Firms must take a comprehensive view, considering how well AI/ML solutions align with the company’s overall business goals, their potential for future scalability, and their ability to deliver sustainable, long-term value. The following key areas should guide firms in assessing whether AI/ML technologies represent a solid investment or pose hidden risks.
Alignment with Business Strategy and Revenue Impact
The most valuable AI/ML implementations are those that directly support the company’s core business strategy. During due diligence, firms should evaluate whether AI/ML technologies are integral to the company’s key revenue streams or simply an experimental or supplementary feature. For instance, is AI/ML driving product differentiation, improving operational efficiencies, or enhancing customer experiences in ways that create a tangible competitive advantage? The closer the alignment between AI/ML solutions and the company’s strategic goals, the greater the likelihood that these technologies will drive sustainable value.
Scalability and Long-Term Potential
Scalability is critical when assessing the value of AI/ML technologies. Firms need to evaluate whether the existing AI/ML infrastructure can support future growth without requiring costly reengineering. This includes assessing whether models and data pipelines are built to handle increased data volumes, more users, or expanded markets. Firms should also consider whether the AI/ML technologies have the flexibility to evolve with the business as its needs change. AI/ML solutions that are adaptable and scalable can continue to provide value as the business grows and markets shift.
Differentiation vs. Commoditization Risk
In evaluating AI/ML capabilities, it’s important to distinguish between what truly provides a competitive advantage and what risks becoming commoditized. While AI/ML technologies may offer differentiation in the short term, advancements in generalized AI could erode that advantage over time. Firms should assess whether the target’s AI/ML assets are unique or rely on proprietary data and models that are difficult to replicate. If these capabilities are vulnerable to being replaced by off-the-shelf solutions, their long-term value may be limited. Understanding how the company plans to stay ahead of commoditization—through continuous innovation or integration of specialized models—will be crucial in determining the real worth of AI/ML investments.
Conclusion
Ultimately, firms that take a strategic and nuanced approach to assessing AI/ML during due diligence will be better positioned to make informed investment decisions. This means evaluating not only the current impact of AI/ML technologies but also their potential to evolve and deliver value as the company scales and as the AI/ML environment shifts. By considering factors such as the team’s expertise, thoughtful model selection, and adaptability, firms can better gauge the true value and risks of AI/ML assets. Focusing on long-term sustainability, governance, and maintaining a competitive edge will ensure that these assets contribute lasting value to the portfolio.
How AKF Can Help
Evaluating AI/ML capabilities during tech due diligence requires a careful balance of technical insight and strategic foresight. At AKF, we specialize in guiding firms through the complexities of assessing AI/ML solutions, from evaluating scalability and security to understanding team maturity. With our deep experience in technology due diligence and AI/ML evaluation, we help you make informed investment decisions that align with your long-term goals. Whether you’re navigating the value of AI/ML in an acquisition or ensuring your current investments deliver sustainable growth, AKF is here to support your journey towards technology-driven success.
Contact AKF to learn more.
Related Posts
</br>